Using artificial intelligence for genomic research
By Katrina Costa, Science Writer, Wellcome Sanger Institute.
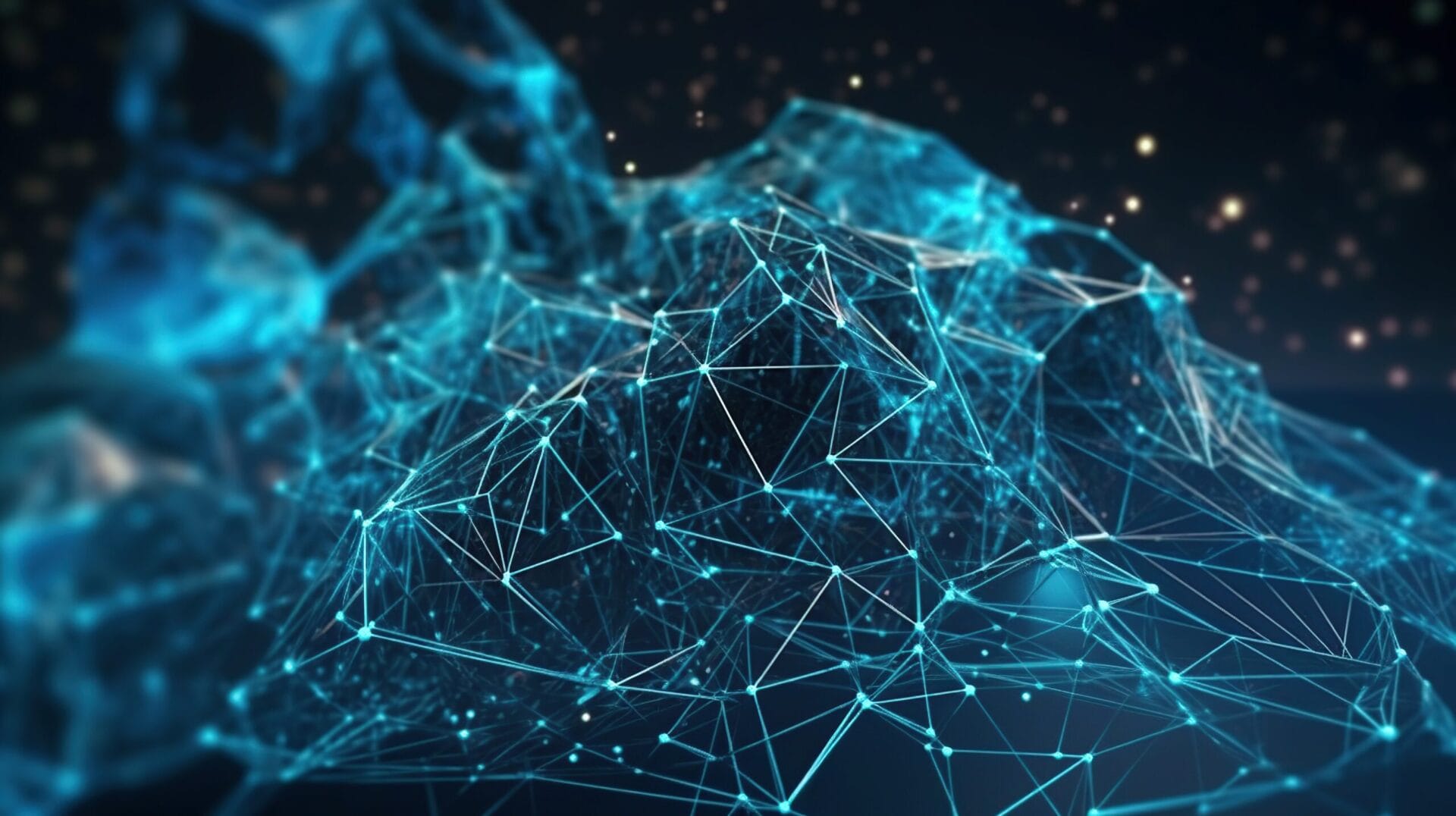
Scientists use artificial intelligence (AI) to improve our understanding of many aspects of biology. Applications range from insights into biological processes, better knowledge of complex diseases or health issues, and tools for engineering new drugs. But what exactly is AI? On this page we break down the key concepts in AI and explain how these technologies help scientists make new discoveries.
Key terms
Artificial Intelligence (AI)
A set of computational tools or machine intelligence that can analyse large data sets to find patterns and connections, and extract meaning.
Machine Learning (ML)
A sub-field of artificial intelligence that can perform classification, prediction and data compression. Machine learning requires training by humans in order to complete any tasks.
Deep Learning (DL)
A sub-field of machine learning. These tools make predictions and decisions using ‘neural networks’ – stacks of connected artificial neurons, inspired by the human brain.
Generative AI (gen AI)
A type of AI that can create content, such as images, text, or even music, most commonly by using deep learning tools. Gen AI has the ability to create ‘new’ data similar to the data it was trained on.
Until recently, most people had only heard about AI in the plots of science fiction movies. But since the launch of Open AI’s ChatGPT, AI has rapidly made its way into our everyday lives. AI is now one of the most exciting fields in modern science and many biological research organisations are embracing AI tools to help unlock information hidden in our DNA and shape the future of genomics and healthcare.
- AI is valuable in genomics because it can enable researchers to analyse vast and complex genomic data more efficiently and accurately than before.
- Machine intelligence can help us with tasks such as reasoning, problem-solving, and understanding patterns.
- Whilst AI holds great promise for genetic research, ethical and social concerns must be addressed.
What is AI?
AI is a broad, cross-disciplinary field that spans computer science, mathematics and statistics, philosophy and cognitive science. Whilst AI lacks a precise definition, it can be viewed as a collection of tools and techniques that provide machine intelligence.
AI can help us with reasoning, problem-solving, and understanding patterns. The tools range from simple rule-based systems to complex learning algorithms.
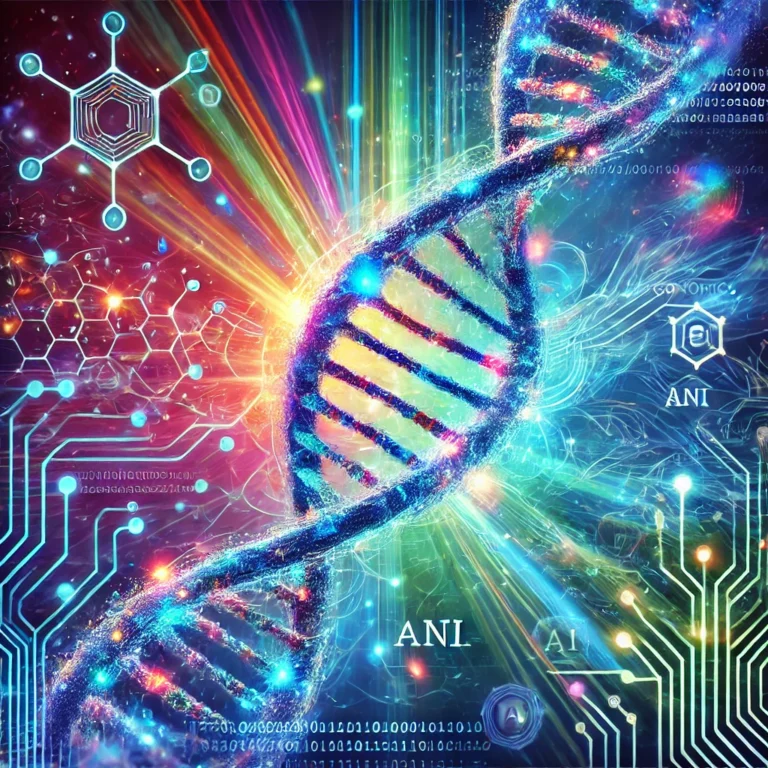
What are the main types of AI?
AI can be loosely split into several sub-types, with much overlap between the categories. The common groupings include machine learning, neural networks, deep learning, and natural language processing.
Machine learning (ML)
ML is a category of AI – so all machine learning is AI, but not all AI involves machine learning.
ML tools use data either from initial training, or gained during experiences, to improve their performance on specific tasks. ML systems improve over time as they are exposed to more data. They can recognise patterns, use intelligence (such as decision-making) and even make predictions.
However, traditional ML tools rely on human guidance to direct the learning process. This is called ‘supervised learning’, which requires large sets of labelled training data.
For example, we could use simple ML to identify emails as ‘spam’ or ‘not spam’. The ML algorithm could be trained on a set of labelled emails and would learn to distinguish between the two categories based on features such as word frequency or email length.
Neural networks
A type of ML model inspired by the structure of the human brain. Neural networks contain nodes (or ‘neurons’) – the fundamental building blocks for processing and transmitting information in a network. These ‘neurons’ are arranged in interconnected layers and adjust the strength (or ‘weights’) of the connections based on the inputs provided by human programmers.
Simple neural networks can be useful for pattern recognition and do not require many layers. For example, we could show the AI a series of labelled pictures (e.g. ‘dog’ or ‘cat’). The AI uses these images to learn the distinguishing features of each animal (like shapes, colours, or sizes) and decide the minimum information it needs to categorise something as a cat or dog. Eventually, it can look at a picture it has never seen before and predict whether it is a cat or a dog.
Deep learning (DL)
A more sophisticated version of ML than the pattern recognition example we explored above. DL uses neural networks stacked into multiple layers hence the word ‘deep’. DL tools can model complex patterns and relationships in data and are used in advanced facial recognition technology of smartphones and security cameras.
DL machines use these complex layers of ‘neurons’ to uncover sophisticated patterns in their training data. The model will collect diverse information from the training data such as light and dark areas, shapes, distance, spatial relationships and so on. The model combines this information in random ways to identify patterns and make decisions.
Deep learning can enable unsupervised learning, which does not require labelled training data. In scientific research increasingly large data sets and complex tasks mean that the unsupervised learning capabilities of DL are becoming important to save time and cost.
Natural Language Processing (NLP)
A sub-type of AI that teaches computers to understand and interact with human language. NLP allows computers to read, listen, and even talk in a human-like manner. To achieve this, the models need to interpret the meaning behind the words so their responses make sense to us.
NLP is used in applications like voice assistants (including Siri and Alexa), chatbots, translation apps, voice recognition, and even grammar-checkers (such as Grammarly).
Generative AI (gen AI)
Gen AI typically uses deep learning tools to create content, such as images, text (including the familiar Large Language Models, LLMs), or even music. Unlike traditional ML models, which make classifications or predictions based on existing data, generative AI is designed to create ‘new’ data similar to the data it was trained on.
There are several types of gen AI, but the most popular early tools for public use (such as Claude and Google Bard) were examples of transformer models. These models train on huge amounts of data to make predictions. For example, Open AI’s GPT (Generative Pre-trained Transformer) uses text data to predict the next word in a sentence. This allows them to create content that makes sense and is presented in the correct context.
However, gen AI can be trained in other ways. For instance, other models use ‘generative adversarial networks’ (GANs). These use two artificial neural networks that train together – a generator (which creates new data, such as an image) and a discriminator (which tries to distinguish real data from those produced by the generator). In this way, GANs create increasingly realistic images. For example, StyleGAN2 from Nvidia can create realistic photos of people that do not exist. A more controversial use case is the creation of ‘deep fakes’ that create images or other media that appear real but are artificially created by a gen AI.
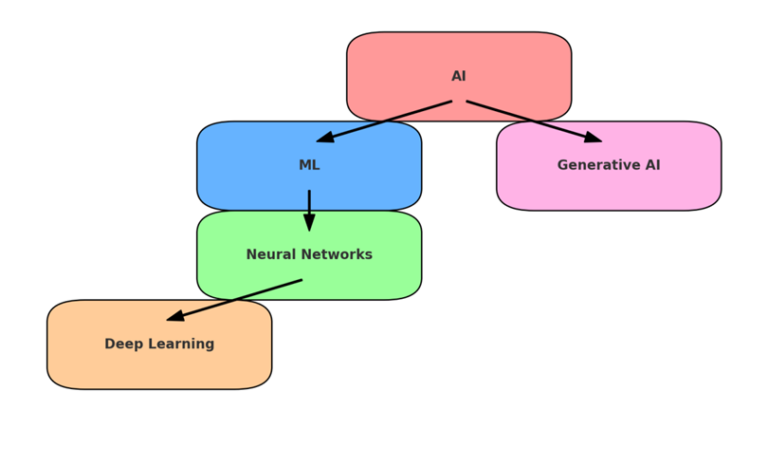
Why is AI useful for genomics?
AI is valuable in genomics because it enables researchers to analyse vast amounts of complex genomic data more efficiently and accurately than before. For example, each human genome contains around 3 billion base pairs. Large-scale studies can involve thousands of different genomes, each containing billions of letters of DNA code, meaning that comparing the letters to find patterns difficult. AI can also help identify patterns and correlations in data that are too subtle or complex for simple analysis to detect. They can also predict the impact of specific changes. But there is a risk of false positives (identifying patterns that are not correct), which means lots of data is required to gain confidence in any findings.
Generative AI could transform the field of genomics by offering innovative tools and approaches to understanding complex biological data. It will become increasingly important as genomic data grows in volume and complexity, and the power of AI tools also continues to grow. This has led to the new scientific field of generative genomics.
What ethical and societal concerns surround AI?
Whilst generative AI is an exciting new field with many potential benefits, including for genomics, there are some ethical concerns. These will require careful and informed discussions with experts from diverse fields, and many organisations are already exploring the impact of AI.
Broadly, the issues cover potential for misuse of AI, bias and fairness, copyright issues and wider societal impacts such as environmental concerns. Notice that most ethical concerns are not restricted to AI, and there are established regulatory frameworks for fields such as science. The arrival of gen AI simply makes the situation more complex, owing to the vast training datasets involved, which are often combined in new and surprising ways. Regulators also can’t predict how AI might innovate in the coming years. Solutions must include high-quality data underlying these AI models, updated regulatory frameworks and oversight, and continuous monitoring of changing technologies and society’s attitudes to AI and genomics.
What’s next for AI in genomics?
AI – especially generative AI – is rapidly advancing into our everyday lives, and will play a crucial role in the future of genomic research. As genomic datasets continue to grow in volume and complexity, AI will become essential for efficiently analysing and understanding these research outputs.
Generative AI could enhance much of the scientific understanding of genomics, including genetic variation, how mutations affect DNA function, and even how to create new genetic sequences. This may bring us closer to personalised medicine. Scientists will apply AI across multiple data types to gain a more thorough understanding of biological processes.
However, as AI tools continue to push technological boundaries, it is essential that research organisations include ethical considerations and equity in the design and delivery of their research, and that governing bodies invest in addressing the social and ethical implications. Responsible and explainable AI is essential for securing public trust and maximising the benefits these tools bring. The Sanger Institute is well-positioned to be a leader in this field, with its large-scale data generation and investment in AI-supported research and the ethics surrounding it.
Images
There are two images in this article that have been generated using Open AI's ChatGPT.
Notice there are some oddities in the images, such as the random letters appearing in the first image and the diagram is not as ‘clean’ as something created with a graphic design programme. These ‘features’ have been intentionally left in to accurately reflect what the model provided us with.
These images are intended as a one-off demonstration of the capabilities of AI when this article was published in 2024. They are for public interest purposes and reflects the content of this article. They are not intended to endorse any tool and YourGenome does not usually use AI-generated imagery.
Image 1: A visual representation of AI in genomics.
This image was created by author Katrina Costa using OpenAI's ChatGPT Plus, version GPT-4o. This image used DALL.E 3, which is integrated into GPT-4o.
Prompt: A conceptual image representing 'the power of AI in genomics'. The image features a large, glowing double helix DNA strand at the center, with dynamic colors such as blues, greens, and purples. Around the DNA strand, there are abstract digital motifs like binary code, circuit patterns, and neural network structures interwoven, symbolizing AI integration. Bright lines connect neural network nodes around the DNA, representing data processing. The background is a vibrant, futuristic digital landscape with a sense of depth, incorporating beams of light and energy to symbolize innovation.
Image 2: Diagram of AI sub-types
This diagram was created by Katrina Costa using OpenAI's ChatGPT Plus, version GPT-4o. The AI used Matplotlib, a visualiser tool based on Python, to generate this diagram.
Prompt:
AI Hierarchy and Relationships: The diagram illustrates the hierarchical relationships between various sub-types of Artificial Intelligence. It starts with AI at the top, branching into sub-categories to depict their interrelationships.
Components and Positioning:
- AI (Artificial Intelligence): Positioned at the top center, marked as the overarching category.
- Machine Learning (ML): Shown as a direct subset of AI, branching downward to the left.
- Neural Networks: Depicted as a subset of ML, further branching downwards from ML.
- Deep Learning: Represented as a specialized form of Neural Networks, branching to the left.
- Natural Language Processing (NLP): Shown as another sub-type of Neural Networks, branching downward to the right.
- Generative AI: Positioned directly under AI but slightly to the right, illustrating that it is a specialized sub-type of AI, alongside ML.
Visual Elements:
- Boxes: Each category and sub-category is represented by a box with rounded corners to give a clean, professional appearance.
- Arrows: Solid arrows connect the boxes, indicating the direction of the hierarchy and the relationship between different sub-types.
- Colors: Different colors are used for each box to visually differentiate the categories: AI (red), ML (blue), Neural Networks (green), Deep Learning (orange), NLP (purple), and Generative AI (pink).
- Spacing: Extra space is added around the arrows and boxes to prevent overlap with text, enhancing readability.
Adjustment Notes:
- The entire diagram is slightly shifted to the right to ensure all curved edges of the boxes are fully visible, and the text within the boxes does not overlap with the arrows.
- The diagram fits within the standard canvas size, making it suitable for presentations and reports.